Data-Driven Healthcare: How Information Saves Lives
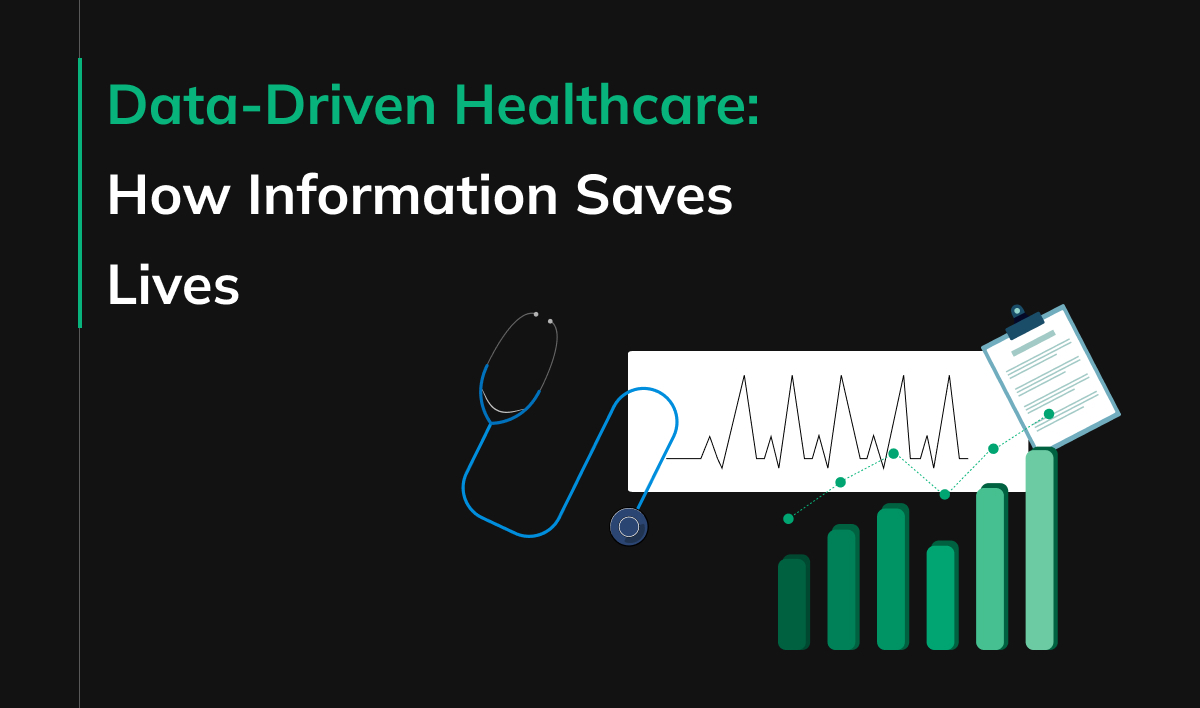
What if we could predict heart attacks before they happen? Well, we’re already doing it! According to the National Institutes of Health, data analytics can reduce hospital readmissions by over 33%. This highlights how data-driven healthcare can save lives.
Data-driven healthcare involves using vast amounts of information to make decisions about patient care. This data comes from various sources, such as electronic health records, wearable devices, and even social media. By analyzing this data, care providers can identify trends, predict outcomes, and personalize treatments. In doing so, they are redefining the modern approach to health and medicine.
In this article, we will explore how data-driven decision-making is revolutionizing the healthcare industry. We’ll discuss its benefits, from improving patient outcomes to reducing costs. We’ll also look at real-world applications, such as predictive analytics and personalized medicine. Through this, you’ll understand why and how information is saving lives.
Key Points
|
The Evolution of Data-Driven Healthcare
Traditionally, healthcare has always used data in some form. Doctors kept detailed patient records and shared medical knowledge through books and journals. For example, patient charts tracked symptoms, treatments, and outcomes, helping doctors make informed decisions. However, this data was often siloed, meaning it was kept in separate, non-digital formats that made it difficult to share and analyze on a larger scale.
Technological Advancements
The real transformation began with the advent of electronic health records (EHRs). EHRs made it easier to store and access patient information digitally, revolutionizing data management. Then came the advancements, such as artificial intelligence and machine learning, which became the backbone of intelligent automation in healthcare. These technologies can process and analyze huge amounts of data much faster and more accurately than humans can. For instance, AI can scan medical images to detect early signs of diseases like cancer, often before a doctor could spot them.
Big data analytics has also played a significant role. This involves examining large sets of data to uncover patterns and trends. In healthcare, this can mean analyzing thousands of patient records to identify risk factors for diseases. Wearable devices, like fitness trackers and smartwatches, add another layer of data. They provide continuous health monitoring, which can alert users and doctors to potential health issues in real-time.
Current Trends
Today, the field of analytics-driven healthcare is booming with innovation. One of the most exciting trends is predictive analytics. By analyzing historical data, we can predict future patient health results. For example, hospitals use this to forecast which patients are at risk of complications after surgery, allowing for proactive interventions.
Another trend is personalized medicine. This approach tailors treatments to individual patients based on their genetic makeup, lifestyle, and other factors. It’s already being used in cancer treatment, where therapies are designed to target specific genetic mutations in a patient’s tumor.
Telemedicine has also surged, especially during the COVID-19 pandemic. It leverages data to provide remote patient care. Through video consultations and digital monitoring, doctors can treat patients without needing an in-person visit. This not only makes healthcare more accessible but also helps in collecting valuable clinical information.
Key Components of Data-Driven Healthcare
Electronic Health Records
EHRs are at the heart of analytics-driven healthcare. They replace paper records with digital ones, making it easier to store, retrieve, and share patient information. EHRs contain comprehensive data about patients, including medical history, diagnoses, medications, treatment plans, immunization dates, allergies, radiology images, and laboratory test results.
For example, if a patient visits a new doctor, their EHR can be quickly accessed to provide a complete medical history, ensuring continuity of care. According to a study by the Office of the National Coordinator for Health Information Technology, 96% of hospitals in the U.S. use EHRs. This widespread adoption helps health service providers make more informed decisions, improving overall public health.
Wearable Devices and IoT
Wearable devices and the Internet of Things play a crucial role in collecting real-time patient data. Devices like fitness trackers, smartwatches, and medical monitors collect data on heart rate, activity levels, sleep patterns, and more. This continuous stream of data helps doctors monitor patients’ health outside of clinical settings.
For instance, wearables can alert users to abnormal heart rates, prompting them to seek medical advice before a condition worsens. A study by the American Heart Association found that wearable technology can help reduce the risk of heart disease by encouraging more physical activity and healthier lifestyle choices.
Cloud Computing
Cloud computing supports the storage, processing, and accessibility of vast amounts of healthcare data. It enables healthcare providers to store patient records, medical images, and other health data securely online. This makes it easier for authorized personnel to access the data from anywhere at any time.
Cloud technology also facilitates the sharing of data between different health service providers, improving collaboration and coordination. For example, a patient treated in one hospital can have their records accessed by a specialist in another city, ensuring they receive the best possible care. Additionally, cloud computing can support large-scale data analysis, helping researchers find new insights and treatment options.
Data Privacy and Security
Ensuring patient data is secure and compliant with regulations is a top priority in data-driven health management. Sensitive health information must be protected from unauthorized access and breaches. Healthcare services must always comply with regulations. These include the Health Insurance Portability and Accountability Act (HIPAA) in the U.S., which sets standards for protecting patient information.
Data encryption, tokenization, secure access controls, and regular security audits are essential for data protection in health systems. For example, a hospital might use encrypted communication channels to transmit patient data and require multi-factor authentication for accessing EHRs. Ensuring robust data privacy and security builds trust with patients and allows care providers to use data confidently to improve care.
Benefits of Data Driven Decision Making in Healthcare
Improved Patient Outcomes
Data-driven healthcare significantly enhances patient outcomes. By using data, doctors can create personalized treatment plans tailored to each patient’s unique needs. For instance, genomic data allows for treatments that target specific genetic mutations in cancer patients, leading to more effective therapies. Predictive analytics is another powerful tool. It uses data to foresee potential health issues before they become severe. For example, by analyzing patient data, hospitals can predict which patients are likely to develop complications after surgery, allowing for early interventions. Studies have shown that predictive analytics can significantly reduce hospital readmission rates [1, 2, 3].
Operational Efficiency
Data science doesn’t just help patients; it also improves how hospitals and clinics run. By streamlining administrative processes, healthcare providers can reduce costs and save time. For example, staff schedules can be optimized with the help of data analytics. This ensures that enough medical professionals are available during peak times without overstaffing during quieter periods. The use of data extends to inventory management as well. Hospitals can track supply usage and predict future needs, preventing shortages of critical items like medications and surgical supplies. A report by Deloitte shows that hospitals using data-driven decision-making can reduce operational costs.
Enhanced Research and Development
Data-driven approaches are revolutionizing medical research. Real-world data from patients helps researchers understand diseases better and develop new treatments faster. For example, analyzing data from thousands of patients can reveal patterns and insights that lead to new discoveries. During the COVID-19 pandemic, data analytics played a crucial role in tracking the spread of the virus and developing vaccines. Companies like Moderna used data to accelerate the development of their mRNA vaccine, a process that traditionally takes years, in just months. This rapid progress is due in large part to the ability to analyze vast amounts of real-world data quickly.
Case Studies: Data-Driven Approach in Action
Several healthcare organizations are leading the way in data-driven healthcare.
Kaiser Permanente, a leading healthcare group, has revolutionized its 39 hospitals and over 700 medical offices in the U.S. by embracing data analytics. By automating processes and analyzing key data points like bed availability and patient flow, they have significantly reduced patient waiting times and eliminated manual data preparation for hospital leaders. This transformation highlights the power of analytics in improving patient care and operational efficiency.
Blue Shield of California has also embraced analytics-driven healthcare. They use data to create personalized health plans for their members, improving overall health outcomes. For example, their data analytics program helps identify members who would benefit from disease management programs, leading to better management of conditions like diabetes and hypertension.
One of our clients faced a unique challenge involving cultural sensitivity. Using data from their existing operational database, we built a platform that integrates patient data with external sources. This platform includes a patient management system tailored to health coordinators and nursing staff, as well as a specialized database focused on ethnic minorities. This data-driven approach helped us deliver culturally competent care. It also improved patient engagement and satisfaction, ensuring no one is left behind due to cultural barriers.
Challenges and Solutions
Data Integration
One of the biggest challenges in data analytics-driven healthcare is integrating data from different systems. Often, hospitals and clinics use different software that doesn’t easily share information. This is called interoperability. To overcome this, healthcare professionals are adopting standardized formats for data sharing. For example, the Fast Healthcare Interoperability Resources (FHIR) standard helps different systems communicate more effectively. Another solution is using middleware—software that connects different systems and allows them to work together seamlessly. By improving interoperability, healthcare providers can ensure that patient data is comprehensive and accessible.
Data Quality
Ensuring the accuracy and reliability of collected data is crucial. Poor data quality can lead to incorrect diagnoses and ineffective treatments. To address this, medical care providers must establish strict data governance policies. This includes regular audits to check the accuracy of data and training staff on best practices for data entry. Advanced technologies like machine learning can also help by identifying and correcting errors in data. For instance, algorithms can detect anomalies that might indicate a mistake, such as an impossible blood pressure reading.
Patient Engagement
Encouraging patients to participate in data sharing is vital for comprehensive care. However, patients might be reluctant due to privacy concerns. To build trust, healthcare providers must be transparent about how data is used and protected. They should educate patients on the benefits of data sharing, such as improved personalized care and better health outcomes. Providing easy-to-use platforms for patients to access and manage their clinical information can also enhance engagement. For example, patient portals allow individuals to view their medical records, schedule appointments, and communicate with their medical care providers.
Addressing Inequalities
Data can help identify and address health disparities, ensuring equitable care for all patients. By analyzing data, medical care providers can spot patterns that indicate inequality, such as certain groups having higher rates of chronic diseases. For instance, data might show that a particular community has a high incidence of diabetes. This information can lead to targeted interventions, like community health programs or mobile clinics, to address the specific needs of that population. Additionally, using data to monitor outcomes can help ensure that these interventions are effective and adjusted as needed.
Future Perspectives for the Healthcare Sector
The future of data-driven healthcare looks promising with exciting advancements on the horizon. Innovative technologies like AI in diagnostics, telemedicine, and remote monitoring are improving patient care by making it more accessible and efficient. Government policies, such as HIPAA and the 21st Century Cures Act, support these changes by ensuring data security and promoting easier data sharing. Emerging trends, including personalized medicine and the integration of social determinants of health, will lead to more tailored and effective treatments. Overall, these developments will create a more efficient healthcare system, offering better outcomes for patients and making healthcare more equitable and comprehensive.
Conclusion
Healthcare evolves rapidly, supported by new technologies and government policies. An increasing focus on personalized medicine and addressing health disparities will further improve health outcomes. Data-driven healthcare isn’t just a technological advancement; it’s a paradigm shift that is saving lives and shaping the future of medicine.