Data science in finance: use cases, trends, and considerations
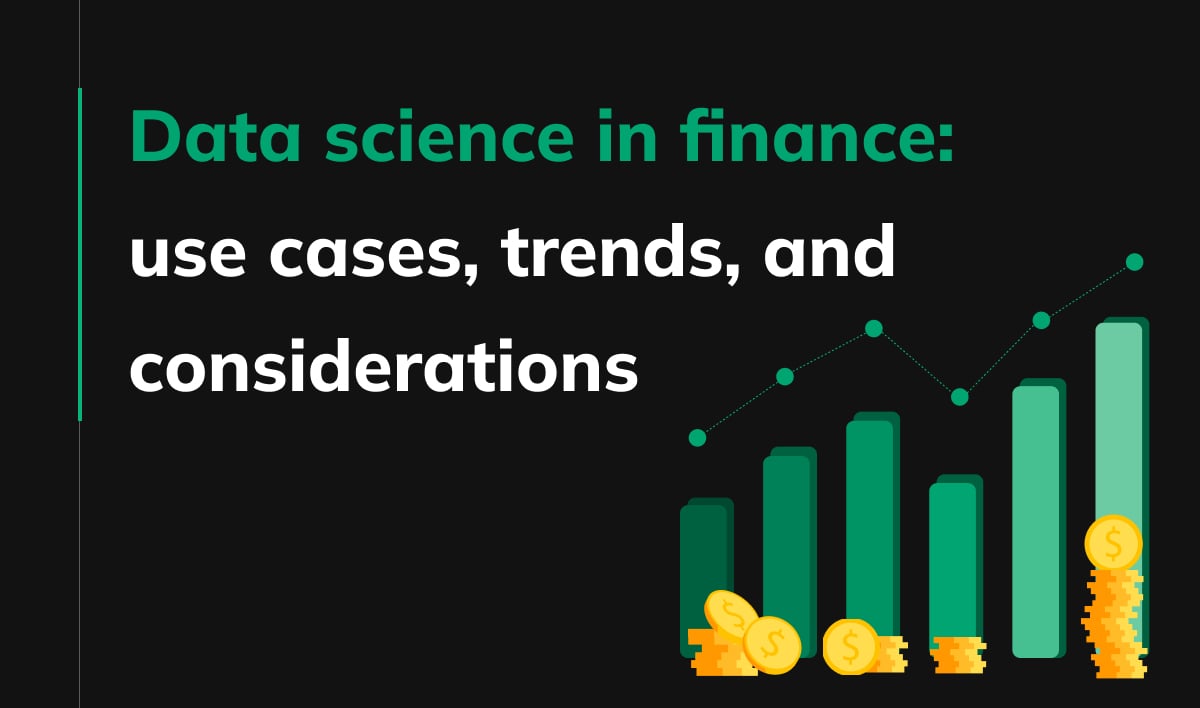
Data science is a blend of statistics, computer science, and business acumen. A data scientist transforms raw data into meaningful insights. In finance, it’s like having a detective to examine the intricate patterns of markets and consumer behaviors.
Now, imagine the finance industry as a vast ocean of customer data; numbers, trends, and transactions. Data scientists are the navigators in this ocean, steering through massive waves of information to find insights. The significance of data science in finance isn’t just growing; it’s skyrocketing. And because of this, today’s financial sector is being rapidly reshaped. It seems that, nowadays, even the mere thought of launching a fintech product necessitates a robust development plan.
Key Points
|
The role of data science in finance
Data rules modern finance. It drives decisions and sparks new ideas. Combined with financial expertise, it reshapes everything from legacy banking to emerging FinTech.
The evolving landscape of finance
Finance moves faster than ever. Each transaction, market signal, and consumer action adds to a massive data pool. Business analytics must adapt quickly to keep up. Regulations are also in flux, forcing banks and FinTech firms to stay compliant while staying competitive.
And there’s FinTech, the (relatively) new kid on the block. It’s shaking up traditional finance with innovative tech and fresh ideas. FinTech firms are like speedboats zipping around larger, slower ships—the traditional banks. They’re changing the game, making everyone rethink how finance works. As professionals who develop financial software, we see this happening right before our eyes.
At the center of this shift are core banking systems. They form the backbone of modern financial institutions. They power smooth operations and enhance customer experiences. (For more details, see our full article on core banking systems.)
Data science in finance: a quick look
Data science has changed the game. It converts raw information into actionable insights. It helps predict trends and understand customers on a deeper level. Skilled engineers in finance do this every day.
Risk management and fraud detection both benefit. Data-driven tools spot threats faster than ever. They strengthen security and prevent losses.
Data science also boosts portfolio optimization. It fine-tunes investments with precision, like having a top-tier strategist on call 24/7.
Above all, data science drives innovation. It positions companies to stand out and evolve. In a crowded market, it’s a true competitive edge.
Core applications of data science in finance
As you can see, data science isn’t just a part of finance; it’s reshaping its very core. Let’s now explore how this tech is revolutionizing key areas of the industry.
Risk analytics and management
In the high-stakes world of finance data analytics, risk management is akin to walking a tightrope. And data science professionals bring a safety net to the table. Predictive analytics is now a go-to tool for assessing market risks and spotting potential crashes before they happen. It’s like having a crystal ball, but backed by hard data.
Machine learning algorithms have transformed risk management. AI is pretty smart at sifting through huge piles of data to spot patterns we’d usually miss. This is a game-changer for predicting risks and making better guesses about what might happen next. It means we can tailor risk plans for each situation and use our resources smarter. Plus, machine learning algorithms take care of the boring, repetitive stuff. They’re always on the lookout, learning and getting better at sniffing out risks early.
Valuation models have also gotten a data science makeover. They’re sharper now, capable of pinning down the fair value of financial instruments with precision. This is crucial for assessing investment risks. Think of this as having a financial GPS guiding you through the fog of market uncertainties.
Fraud detection
Fraud detection has transformed into a sophisticated game of cat and mouse, with data science powering the chase. Transaction analysis now goes deep, picking out patterns and anomalies that scream ‘fraud’. It’s like having a finely-tuned radar scanning for financial mischief.
Machine learning is a game-changer in fraud detection, too. It flags suspicious transactions, sometimes even before they’re completed. These algorithms are like vigilant guardians, always on high alert for signs of fraud.
Predictive models also play a key role. They assess the likelihood of fraud, helping institutions prioritize investigations. It’s a bit like weather forecasting, but for financial storms, giving a heads-up on where to focus the fraud-fighting efforts.
Portfolio management and algorithmic trading
Portfolio management is no longer just an art; it’s a science. Risk-adjusted return models act as precise business intelligence tools. They help plan investment strategies that aim for good returns without ignoring the risks. It’s about making smart, balanced choices for your investments. Think of it as a finely balanced scale, weighing risk against reward.
Machine learning algorithms have a nose for value. They can sniff out undervalued assets and hidden market opportunities. It’s like having a treasure map, revealing where the financial ‘gold’ is buried.
Algorithmic trading systems have revolutionized how trades are executed. They do it automatically, swiftly, and effectively, precisely slicing through market noise. This not only improves efficiency but also cuts down on the risk. The integration of reinforcement learning is pushing these systems even further. This technique allows them to learn and adapt from past trades, almost like gaining experience. They make decisions not just based on preset rules, but by evaluating the outcomes of their previous actions. It’s a step towards making these systems even more intuitive and effective, continuously improving their trading strategies.
New applications of data science in finance
We’ve seen how data science is a game-changer in risk management, fraud detection, and portfolio management. Now, let’s turn our attention to other areas in finance, where data science is unlocking new opportunities.
Customer segmentation and personalized services
Customer segmentation isn’t just about grouping people anymore; it’s about understanding them and offering them personalized services. Data-driven segmentation slices through demographics, preferences, and behaviors to identify distinct customer groups. This isn’t just sorting; it’s almost like mind-reading.
Targeted marketing campaigns are getting a facelift with this approach. By reaching specific customer segments, companies enhance engagement and build stronger relationships. It’s like having a conversation, but you already know what interests your audience.
Hyper-personalization is key in today’s finance world. Products and services are now tailored to individual needs, thanks to data science. Imagine walking into a store where everything is handpicked just for you. That’s what data science does for customers in finance.
Price optimization
Gone are the days of one-size-fits-all pricing. Data analysis now enables dynamic pricing strategies for different products and services. Data-based dynamic pricing models are the new norm. They’re not just about adjusting prices; they’re about being smart and responsive to the market’s heartbeat. This approach maximizes profit margins and revenue generation. It ensures that companies stay ahead of the curve.
It doesn’t stop there; consider predictive pricing algorithms. They’re the crystal balls of the finance world. By analyzing data, they predict future demand and adjust prices just right. It’s about striking a balance, ensuring companies don’t end up with too much or too little stock.
Regulatory compliance
In the finance sector, staying within the lines of regulation is crucial. RegTech solutions that incorporate data science step in as a powerful ally here. They help identify potential compliance risks through detailed data analysis and risk assessment. It’s like having a high-tech radar constantly scanning for regulatory storm clouds.
Real-time monitoring of regulatory compliance is now possible with compliance dashboards. These dashboards are like the control centers, providing a clear view of where a company stands in terms of compliance.
Finally, there’s the human element. Compliance training programs, enhanced by data science, are equipping employees with better understanding and tools. This helps prevent violations. It’s not just about following rules; it’s about understanding why they matter.
Ethical considerations and societal impact
Data science in finance unlocks immense potential. It can reshape entire markets and empower consumers. Yet it also raises serious questions about ethics and community well-being.
Data privacy and protection
Financial data is a prized resource, demanding robust tools and strict security practices. Encryption and tokenization help guard against threats. Clear disclosures build trust, allowing individuals to understand why their data is collected and how it benefits them. This transparency is a key driver of customer loyalty. Notably, 75% of the global population may be covered by privacy regulations by 2025. This underscores a growing focus on data protection worldwide.
Responsible AI and algorithmic bias
AI can reveal hidden opportunities. But it can also mirror existing biases. Skewed models might deny loans or raise interest rates for certain groups. Regular audits are key to spotting unfair trends. Training on balanced datasets helps reduce bias. Tools like explainable AI bring clarity and build trust. With careful oversight, we can design fair financial products. Yet concerns remain. For example, the UK’s Financial Conduct Authority warns that AI in insurance could make some people “uninsurable” or lead to discrimination.
Social responsibility and financial inclusion
Many people lack access to credit or financial services. Data science can help close these gaps. Micro-lending platforms, powered by smart data analysis, can reach those with limited credit history. This boosts financial inclusion. Yet complex tools often overwhelm newcomers. That’s why education is key. Short, user-friendly resources teach basic finance and digital skills, helping people make smarter money decisions.
Still, there is a risk of bias. AI could mirror social inequalities or exclude certain groups. Industry leaders warn that if left unchecked, such biases could deepen divides. Ongoing oversight and balanced data are crucial. With these safeguards, data science can create fair financial products that lift entire communities.
Data science in finance: future directions and trends
As we enter 2025, data science in finance is evolving at record speed. Technology is driving this surge, and the need for advanced analytics is higher than ever. Below are some key trends to watch.
Advanced machine learning and deep learning techniques
Financial institutions are turning to advanced machine learning (ML) and deep learning (DL) to sharpen decisions in risk management and customer service. The European Banking Authority (EBA) is testing models like random forests and neural networks to detect regulatory breaches more quickly.
In investment management, hedge funds use AI to stay ahead. Man Group, which handles about $175 billion in assets, built ArcticDB to analyze massive tick data from stock trades. This tool speeds up decision-making and showcases the power of data-driven insights. Meanwhile, deep learning approaches—such as Convolutional Neural Networks (CNNs) and Long Short-Term Memory (LSTM) models—help traders spot patterns in large datasets, improving market trend predictions.
Big data and real-time analytics
Big data and real-time analytics are reshaping finance. The ability to process huge amounts of information in milliseconds is now essential. For example, the above-mentioned ArcticDB can handle over 400 billion market ticks daily, enabling fast time-series analysis. This speed is crucial for high-frequency trading, where every second counts.
Fraud detection also benefits from real-time analytics. Banks use predictive tools to monitor transactions and flag risks before they escalate. Cloud-based solutions boost these efforts, offering scalable computing power for continuous data processing.
Open data and collaborative ecosystem
Open data is transforming finance by encouraging innovation and inclusivity. Open banking in the UK and Europe forces banks to share customer data—with consent—so third-party providers can craft new services. This opens the door to personalized products and better customer experiences.
In the United States, new rules from the Consumer Financial Protection Bureau (CFPB) let people transfer personal data between fintechs and banks at no cost. This shift promotes competition and empowers consumers. Open-source data science tools further foster collaboration. They let professionals share insights and build solutions together, creating a more inclusive and efficient financial ecosystem.
The future of data science in finance is taking shape. Advanced ML and DL techniques are driving change. Real-time analytics is becoming essential. Open data and collaboration are gaining momentum. Together, these trends will create a more dynamic, inclusive, and innovative financial landscape.
Conclusions
We’ve taken a deep dive into how data science is transforming finance. It’s a journey from number crunching to shaping entire financial strategies. Data science isn’t just a tool; it’s a storyteller, turning data into decisions, insights, and foresight.
Yet, with power comes responsibility. As we innovate, ethical and societal impacts can’t be ignored. It’s about balancing cutting-edge tech with fairness and care.
Looking forward, the horizon is lit with advanced tech and collaborative potential. But as we step into this bright future, we must walk wisely, blending innovation with integrity, and progress with inclusivity.
In this blend of data and finance, one thing’s certain: change is constant, fast, and full of possibilities.