How to Predict Customer Behavior With AI in 2025
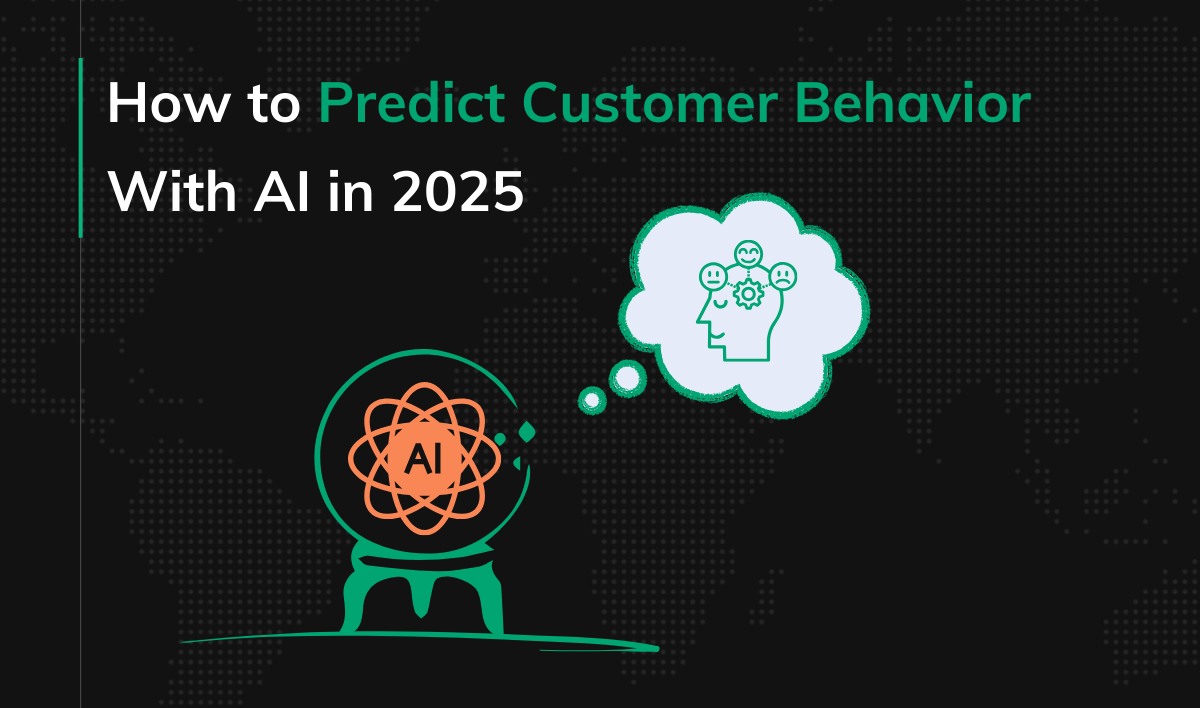
Understanding and predicting customer behavior remains essential for business success. AI is already a crucial asset in predictive analytics. And it is set to become even more critical for customer behavior prediction in 2025. No longer just a tech buzzword, AI is now a practical tool transforming customer insights. In this article, we examine how AI enhances customer behavior prediction and what this means for businesses striving to maintain a competitive edge.
Key Points
|
Understanding Customer Behavior
Grasping customer behavior goes beyond analyzing what people buy; it’s about understanding why they make those purchases. AI brings a new depth to this understanding by processing vast amounts of data to reveal patterns and trends previously hidden from view.
AI doesn’t just look at sales figures. It examines social media interactions, browsing history, and customer feedback. This holistic analysis provides businesses with a fuller picture of their customers. With AI, companies receive real-time insights into customer preferences, allowing them to adapt quickly to changing demands.
Hyper-personalization is another significant benefit. AI enables companies to tailor experiences for individual customers, enhancing satisfaction and building loyalty. By leveraging AI, businesses gain insights into factors that influence human behavior:
- Emotions and Psychology: Feelings and moods can drive purchasing decisions.
- Social Proof: Recommendations and reviews impact choices.
- Cultural Norms: Cultural backgrounds affect preferences.
- Personal Values: Ethical considerations like sustainability influence buying habits.
- Economic Conditions: Personal finances and broader economic trends play significant roles.
- Technological Comfort: Familiarity with technology shapes how customers interact with digital platforms.
Understanding these factors helps businesses anticipate needs and tailor their offerings accordingly.
Predictive Behavior Modeling in Marketing
Predictive behavior modeling uses AI to forecast customer actions, enhancing marketing efforts. It applies advanced algorithms to historical data, uncovering patterns that predict future behavior. This approach improves the accuracy of predictions and helps businesses make informed decisions.
For example, AI can predict customer churn by identifying signs of dissatisfaction, allowing companies to intervene before losing customers. It estimates customer lifetime value, helping prioritize resources for high-value clients. AI also suggests products that complement past purchases, increasing sales through cross-selling and upselling.
Techniques like Bayesian probabilistic models and logistic regression become more powerful with AI, improving both accuracy and efficiency.
- Bayesian probabilistic models use probability to make predictions based on prior knowledge and new evidence. They continuously update predictions as more data becomes available, allowing for more flexible and adaptive forecasting.
- Logistic regression is a statistical method used to predict the probability of a certain event occurring, such as whether a customer will make a purchase or not. It helps classify customers into different categories based on their behavior patterns.
By integrating AI, these techniques can process larger datasets and identify subtle patterns that traditional methods might miss. This leads to more accurate models and better prediction of customer behaviors, enabling businesses to tailor their strategies effectively.
What Influences User Behavior?
Several elements shape how customers make decisions:
- Demographics: Age, gender, income, and education level affect purchasing habits.
- Psychographics: Values, beliefs, interests, and lifestyles offer deeper insights into motivations.
- Social Factors: Family, friends, and social networks influence buying decisions.
- Cultural Influences: Traditions and societal norms impact preferences.
- Economic Conditions: Economic stability and individual purchasing power play significant roles.
- Technological Adoption: Comfort with technology affects how customers interact with businesses.
Understanding these influences isn’t just about adapting to customer needs. It’s about spotting unseen opportunities.
How AI Decodes Influences on Customer Behavior
Analyzing Social Media Trends
AI excels at deciphering complex influences on customer behavior. For example, tools like Brand24 scan social media platforms to detect emerging trends and sentiments, providing valuable insights into public opinion. By analyzing mentions, hashtags, and user engagement, businesses can gauge customer perceptions in real-time. Additionally, by incorporating data on economic indicators like inflation and unemployment rates, AI helps predict spending patterns.
While social media trends might appear obvious, AI can spot these trends earlier and with greater accuracy. This gives businesses a significant advantage. AI algorithms process vast amounts of data in real-time. They detect subtle shifts in consumer sentiment that manual analysis might miss. For instance, if AI notices a surge in discussions about sustainable products, it signals a growing customer preference for eco-friendly options. By identifying these trends ahead of competitors, businesses can promptly adjust their strategies. This allows them to seize new market opportunities and better meet customer demands.
Beyond Social Media: Understanding Cultural Dynamics
AI usage doesn’t stop at social media; AI models can pull data from various sources to understand changing cultural dynamics. Online forums, news articles, and emerging trends across different regions are a goldmine for insights. By aggregating these sources, AI identifies shifts in consumer attitudes and values. For example, a growing interest in remote work technologies might reflect broader changes in work culture.
Tracking how customers use technology offers insights into their preferences and comfort levels with digital platforms. AI analyzes user interactions with apps, websites, and devices. It looks at which features are most used and which are ignored. This reveals not only what customers prefer but also how they adapt to new technologies.
By understanding these nuances, businesses can tailor their products and services to align with evolving cultural and technological trends. They can improve user experience by simplifying interfaces or adding features that match customer expectations. This proactive approach helps companies stay relevant in a rapidly changing market.
Benefits of Predicting Customer Behavior with AI
Leveraging AI for customer behavior prediction offers numerous advantages.
Improved Customer Satisfaction
Personalizing experiences based on predictive insights leads to happier customers. AI suggests products or services that align with individual preferences, increasing relevance. Websites and apps adapt their interfaces and content to suit user behavior. Predictive analytics anticipate issues before they arise, allowing businesses to address them promptly. Focusing on individual needs helps build stronger relationships and fosters loyalty.
Effective Marketing Strategies
Targeted campaigns become more precise, increasing engagement and conversion rates. AI analyzes customer data to deliver highly relevant marketing messages. Resources are allocated to channels and audiences with the highest predicted return on investment. Marketing materials are tailored to resonate with specific customer segments, reducing wasted efforts and maximizing impact.
Better Retention
Anticipating needs aids in developing loyalty programs that keep customers returning. AI identifies patterns indicating a customer is likely to leave, enabling preemptive action. Rewards and incentives are customized to individual preferences and behaviors. AI suggests the best times and methods to reach out to customers, enhancing engagement. Retaining existing customers is often more cost-effective than acquiring new ones.
Increased Sales
Understanding purchasing patterns uncovers opportunities for upselling and cross-selling. AI predicts what customers are likely to purchase next and suggests appropriate offers. Prices adjust in real-time based on demand, customer behavior, and market conditions. Predictive models forecast demand, helping businesses manage stock levels effectively. These insights directly contribute to revenue growth and profitability.
Enhanced Decision-Making
AI tools process large datasets to provide actionable insights, enabling businesses to make data-driven decisions. Predictive analytics forecast future trends and customer behaviors with high accuracy. AI evaluates potential risks in marketing and sales strategies. It provides insights that inform long-term business plans, product development, and market expansion. Data-driven decisions reduce uncertainty and improve business outcomes.
Challenges in Predicting Customer Behavior with AI
Despite the advantages, several challenges must be addressed.
Data Privacy Concerns
Collecting and analyzing customer data raises ethical and legal issues. Compliance with regulations like GDPR is essential to protect customer information and maintain trust. Even when using publicly available data, privacy concerns can arise. Companies can face scrutiny for aggregating and using public data without explicit consent. Monitoring social media platforms like Reddit and X for general insights is generally legal, as long as no personal data is involved. However, businesses must tread carefully to avoid violating terms of service or privacy laws. Transparency and ethical data practices are crucial to maintain customer trust and avoid legal complications.
Inaccurate Data
Poor data quality can lead to incorrect predictions. Ensuring data accuracy through proper cleaning and validation is crucial. Sometimes, there’s not enough data to make accurate predictions, especially in new markets or with niche products. Additionally, vocal minorities on social media can distort the overall perception, leading to skewed insights. Relying solely on social media data might not provide a complete picture of customer behavior. It’s important to combine multiple data sources and use robust statistical methods to mitigate these issues.
Changing Behaviors
Rapid shifts in consumer preferences require adaptable models. AI systems must be flexible and continuously updated to stay relevant. Seasonal changes and trends must be accurately mapped and accounted for. While these patterns are often well-known, models need optimization to incorporate them effectively, improving prediction accuracy. Failing to adjust for seasonal variations can lead to misguided strategies and missed opportunities. Continuous monitoring and model retraining are essential to keep up with changing behaviors.
Limited Resources
Implementing advanced AI systems can be resource-intensive, requiring investment in technology and skilled personnel. Not all businesses have the budget or expertise to develop in-house AI solutions. This is where Software as a Service (SaaS) platforms can help. SaaS providers offer scalable AI tools that businesses can use without significant upfront costs.
Overcoming these challenges involves adopting ethical data science practices, investing in data quality management, and choosing scalable AI solutions that can grow with the business.
Steps to Predict Customer Behavior Using AI
Implementing custom AI for behavior prediction involves a structured approach. Here’s a step-by-step guide to harnessing AI effectively. But first…
A Key Consideration: Should You Build or Buy AI Solutions?
Incorporating a ready-made AI solution is often more practical and cost-effective than developing your own models. Many businesses find that existing platforms meet their needs without the hefty investment in technology and talent. Unless your company operates in a very niche context or has both the funds and a solid reason for custom development, it’s advisable to leverage existing tools. For example, a specialized financial institution dealing with unique data might build proprietary models. But for most, ready-made solutions offer ample capabilities.
Implementing Custom AI for Behavior Prediction
1. Data Collection and Integration
Gather data from various sources:
- Transactional Data: Purchase history, cart abandonment, and return rates.
- Behavioral Data: Website navigation patterns, click-through rates, and time spent on pages.
- Demographic Data: Age, location, income level, and education.
- Psychographic Data: Interests, values, and lifestyles collected through surveys or social media.
Integrate this data into a centralized system to ensure seamless analysis.
2. Data Cleaning and Preprocessing
Prepare the data for analysis:
- Remove Duplicates: Eliminate redundant entries.
- Handle Missing Values: Address gaps using methods like imputation.
- Normalize Data: Ensure consistency in formats and units.
Remember, quality data is the foundation of accurate predictions.
3. Feature Extraction
Identify significant attributes that influence behavior:
- Behavioral Attributes: Frequency of purchases, average spend, product preferences.
- Engagement Metrics: Interaction with emails, app usage, response to promotions.
Use feature engineering techniques to create variables that enhance model performance.
4. Model Selection and Creation
Choose appropriate AI models:
- Machine Learning Algorithms: Decision trees, random forests, support vector machines.
- Deep Learning Models: Neural networks for complex pattern recognition.
- Predictive Analytics Tools: Software with built-in algorithms and user-friendly interfaces (check the tools section below).
Train the models using historical data and validate their accuracy.
5. Customer Segmentation
Group customers based on predicted behaviors:
- RFM Segmentation: Categorize by Recency, Frequency, and Monetary value.
- Behavioral Segmentation: Group by product preferences, brand loyalty, or engagement level.
- Dynamic Micro-Segmentation: Use AI to create highly specific customer groups for personalized targeting.
Segmentation allows for more precise marketing strategies.
6. Prediction and Analysis
Run the models to predict future behaviors:
- Churn Prediction: Identify customers at risk of leaving.
- Lifetime Value Estimation: Forecast the long-term value of customer relationships.
- Purchase Propensity: Determine the likelihood of customers buying specific products.
7. Strategy Implementation
Use the predictions to inform business decisions:
- Personalized Marketing: Craft messages that resonate with individual customer segments.
- Product Recommendations: Suggest items based on predicted interests.
- Resource Allocation: Focus efforts on high-value customers or those at risk of churning.
Implementing these strategies enhances customer satisfaction and drives growth.
8. Continuous Monitoring and Improvement
Regularly update models and strategies:
- Monitor Performance: Track the accuracy of predictions and adjust models as needed.
- Incorporate Feedback: Use customer responses to refine approaches.
- Stay Updated: Keep abreast of technological advancements and market trends.
AI Tools for Behavior Prediction
Several AI-powered tools assist in predicting customer behavior.
- Predictive Analytics Software: Platforms like IBM Watson and SAS Customer Intelligence 360 offer robust modeling capabilities.
- User Behavior Analytics Platforms: Tools such as Mixpanel and Amplitude analyze user interactions to identify patterns.
- Real-Time Customer Profilers: These provide instant insights, enabling immediate action. Tools, like Adobe Experience Platform and Algonomy’s Real-Time Customer Data Platform offer customer profiling in real-time.
- Enhanced E-commerce Tracking: Google Analytics offers features to monitor online shopping behaviors.
These tools often integrate with existing systems, making it easier for businesses to adopt AI solutions without overhauling their infrastructure.
Choosing the Right Tool
Consider the following when selecting a tool:
- Scalability and Cost: Can it handle growing data volumes, and is it within your budget? Providers offer varying plans to accommodate different business sizes and needs.
- Ease of Use: Does it require specialized knowledge? Usually, SaaS tools are optimized for user experience, but some advanced features might require specialists to operate.
- Integration Capabilities: Will it work with your current systems? Providers often offer simple integrations with many popular platforms. Also, tools like Zapier can facilitate connections between different systems.
Selecting the right tool ensures you get the most value from your investment.
Conclusion
Predicting customer behavior with AI in 2025 is becoming increasingly important for staying competitive. By understanding and leveraging AI technologies, companies can anticipate customer needs, optimize strategies, and drive growth.
As AI technology advances, its role in customer behavior prediction will evolve significantly. For businesses, this presents new challenges but, more importantly, new opportunities for growth and innovation. For the average user, this means greater convenience, relevant offerings, and a more enjoyable shopping experience.